Transform
팁
Getting your point cloud ready for your application 웨비나에서는 부분적으로 Transform을 다룹니다.
Introduction
이번 자료는 포인트 클라우드 컨텍스트의 변환, Zivid 포인트 클라우드를 변환하는 방법 및 이것이 왜 중요한 기능인지 설명합니다. 마지막으로 코드 예제가 포함된 튜토리얼을 제공합니다.
Zivid 포인트 클라우드는 Zivid camera coordinate system 를 기준으로 제공됩니다. 다양한 이유로 다양한 응용 프로그램은 전체 포인트 클라우드 또는 해당 세그먼트(예: ROI 또는 선택 포인트)를 카메라 좌표계에서 다른 좌표계로 변환해야 합니다.
Problem |
포인트 클라우드는 카메라 좌표계를 기준으로 제공되지만 응용 프로그램은 다른 좌표계를 기준으로 하는 포인트 클라우드가 필요합니다. |
Solution |
Zivid 포인트 클라우드를 카메라 좌표계에서 다른 좌표계로 변환합니다. |
Transform in API
A rigid transformation is defined by a 4×4 homogeneous transformation matrix that describes the pose of one coordinate system relative to another. Once the transformation matrix is known, we can use Zivid SDK to transform the point cloud from the camera coordinate system to the desired coordinate system.
팁
Zivid SDK는 Non-rigid Transformation를 포함하여 모든 affine transformations 을 허용합니다. Non-rigid Transformation의 예는 다음과 같습니다. scaling the point cloud by converting it from mm to m .
As an example, say you have detected the Zivid calibration board and estimated its pose.
std::cout << "Detecting and estimating pose of the Zivid checkerboard in the camera frame" << std::endl;
const auto detectionResult = Zivid::Calibration::detectCalibrationBoard(frame);
const auto cameraToCheckerboardTransform = detectionResult.pose().toMatrix();
std::cout << "Camera pose in checkerboard frame:" << std::endl;
const auto checkerboardToCameraTransform = cameraToCheckerboardTransform.inverse();
Console.WriteLine("Detecting and estimating pose of the Zivid checkerboard in the camera frame");
var detectionResult = Detector.DetectCalibrationBoard(frame);
var cameraToCheckerboardTransform = new Zivid.NET.Matrix4x4(detectionResult.Pose().ToMatrix());
Console.WriteLine("Camera pose in checkerboard frame:");
var checkerboardToCameraTransform = cameraToCheckerboardTransform.Inverse();
print("Detecting and estimating pose of the Zivid checkerboard in the camera frame")
detection_result = zivid.calibration.detect_calibration_board(frame)
if not detection_result.valid():
raise RuntimeError(f"No Checkerboard detected. {detection_result.status_description()}")
camera_to_checkerboard_transform = detection_result.pose().to_matrix()
print("Camera pose in checkerboard frame:")
checkerboard_to_camera_transform = np.linalg.inv(camera_to_checkerboard_transform)
This transformation matrix can then be used to transform the point cloud to the checkerboard coordinate system.
std::cout << "Transforming point cloud from camera frame to checkerboard frame" << std::endl;
pointCloud.transform(checkerboardToCameraTransform);
You can save the transformation matrix to file, and also load it from file.
const auto transformFile = "CheckerboardToCameraTransform.yaml";
std::cout << "Saving camera pose in checkerboard frame to file: " << transformFile << std::endl;
checkerboardToCameraTransform.save(transformFile);
The point cloud is transformed in-place, meaning you can save the transformed point cloud to file from the frame.
const auto checkerboardTransformedFile = "CalibrationBoardInCheckerboardOrigin.zdf";
std::cout << "Saving transformed point cloud to file: " << checkerboardTransformedFile << std::endl;
frame.save(checkerboardTransformedFile);
Similarly, you can also load a transformed point cloud. To recover the original point cloud, you can read out the applied transformation matrix to transform it back again.
std::cout << "Reading applied transformation matrix to the point cloud:" << std::endl;
const auto transformationMatrix = pointCloud.transformationMatrix();
std::cout << transformationMatrix << std::endl;
참고
Zivid SDK의 Transform API는 포인트 클라우드 데이터가 GPU 메모리에 있는 동안 GPU에서 병렬로 수행되기 때문에 속도가 빠릅니다. 타사 라이브러리를 사용한 변환 구현은 시간이 더 많이 소요될 수 있습니다. 일반적으로 CPU 계산은 훨씬 느리고 GPU 계산에는 다른 복사본이 필요합니다. 보다 많은 정보를 확인 하고 싶다면 Point Cloud Capture Process 을 확인해주세요.
팁
For better performance, use the transform API together with the downsample and normals APIs. This ensures that all computations are performed by the GPU while the point cloud data remains in GPU memory.
가장 빠른 구현을 위해 먼저 포인트 클라우드를 다운샘플링한 다음 변환합니다.
포인트 클라우드와 동일한 좌표계의 노멀을 갖도록 포인트 클라우드를 먼저 변환한 후 노멀을 계산합니다.
포즈(Poses)와 변환(Transformations)에 대해 자세히 알아보려면 다음을 확인하세요. Position, Orientation and Coordinate Transformations.
Transform in Zivid Studio
You can also apply transformations to the point clouds in Zivid Studio. After having captured, navigate to the Transform drop-down at the top of the current view. Among others, you can load a transformation matrix from file, set it to the coordinate system of the chosen ROI Box, and save the transformation matrix to file.
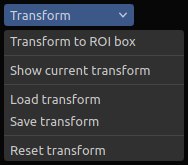
Transform drop-down menu in Zivid Studio
This allows you to visualize transformed point clouds in Zivid Studio, which can be useful when evaluating the point cloud quality. It also allows you to transform the point cloud to a chosen coordinate system, and save or export the transformed point cloud to file.
Point clouds are by default given in the camera coordinate system as in (a), but you can transform the point cloud to a more suitable coordinate system as in (b).
Transform in applications
이 섹션에서는 포인트 클라우드 변환을 활용하는 Zivid 카메라 및 로봇 애플리케이션을 다룹니다. 이러한 응용 프로그램의 일반적인 좌표계와 포인트 클라우드를 이러한 좌표계로 변환하는 것이 유용한 이유를 설명합니다.
Picking
로봇이 포인트 클라우드에서 감지된 물체를 선택하려면 좌표가 카메라 좌표계에서 로봇이 이해할 수 있는 좌표계로 변환되어야 합니다.
Robot coordinate system
우리는 종종 객체 좌표 또는 전체 포인트 클라우드를 로봇 기본 좌표계로 변환하고 싶습니다. 이것은 기사에 자세히 설명되어 있습니다 How To Use The Result Of Hand-Eye Calibration.
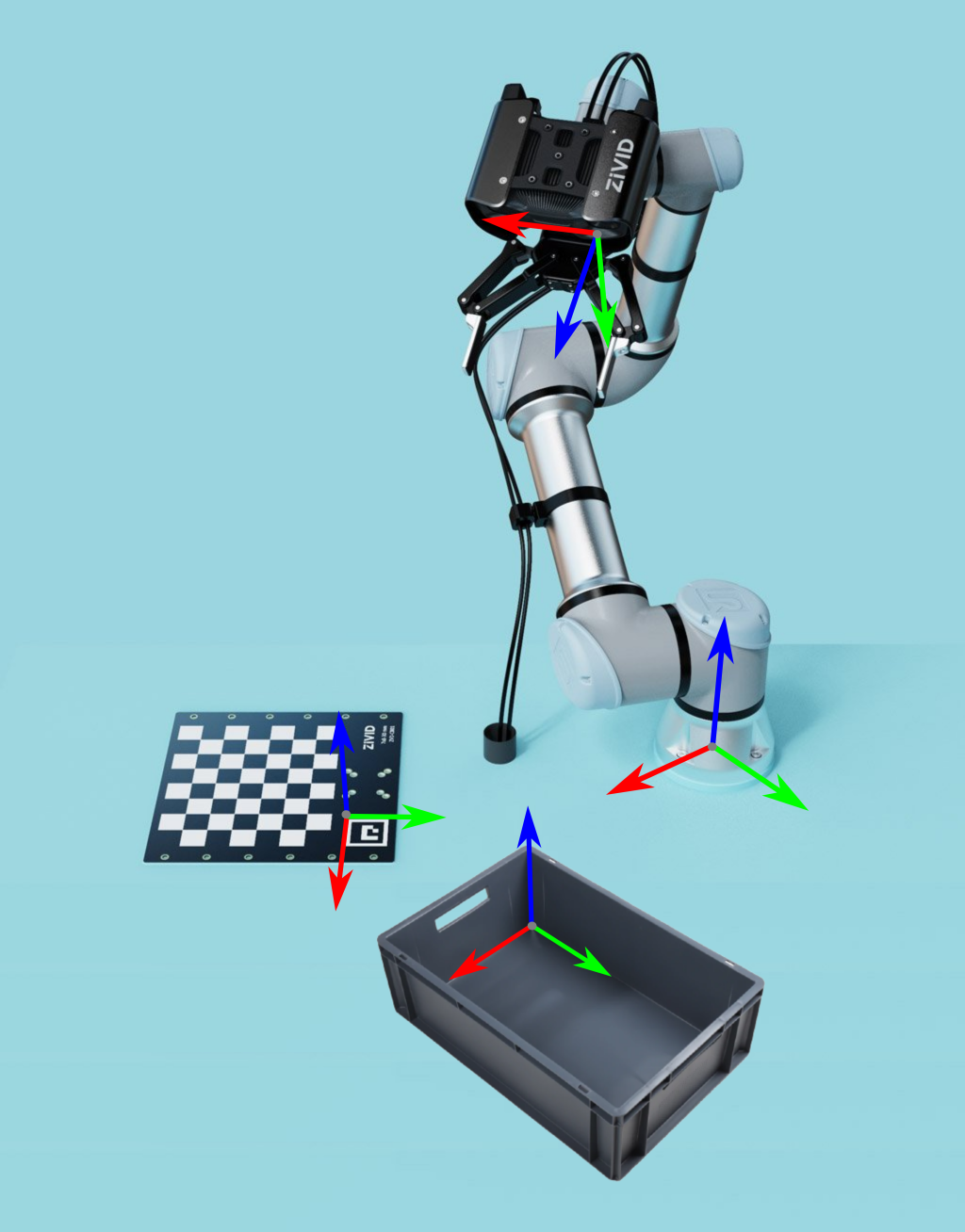
Plane coordinate system
카메라는 종종 피킹 표면과 평행하지 않습니다. 포인트 클라우드 z축이 평면 z축과 일치하도록 포인트 클라우드를 변환하면 포인트 클라우드 데이터를 더 잘 이해하고 해석하는 데 도움이 됩니다. 따라서 포인트 클라우드를 빈 바닥이나 컨베이어 벨트와 같은 피킹 표면의 좌표계로 변환하는 것이 일반적입니다.
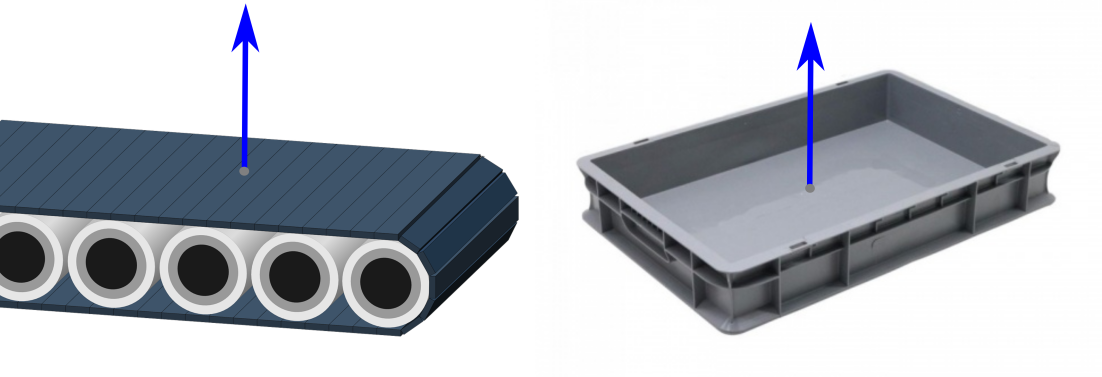
Bin coordinate system
포인트 클라우드 데이터를 더 잘 이해하고 피킹 성능을 평가하는 데 도움이 되는 또 다른 단계가 있습니다. 즉, 포인트 클라우드의 x 및 y 축을 bin의 x 및 y 축과 정렬하는 것입니다.
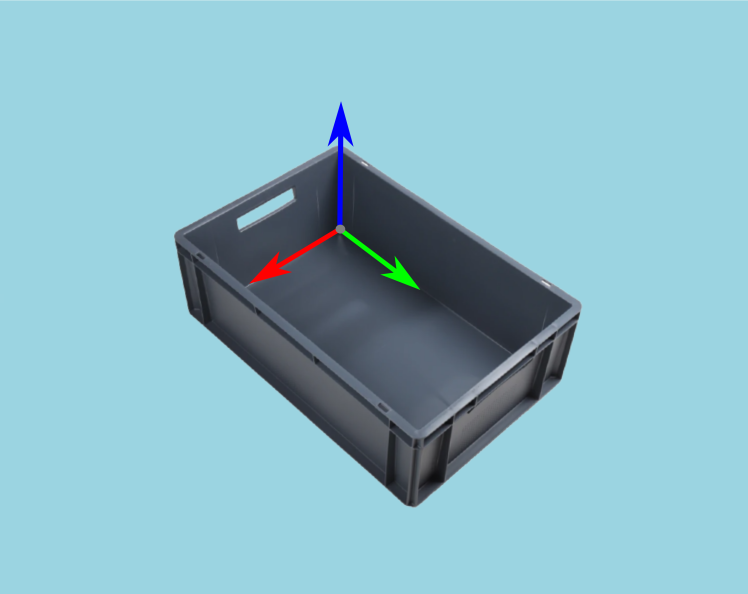
Region of Interest
카메라 시야는 종종 우리의 관심 영역(예: 빈)보다 큽니다. 따라서 빈 축을 포인트 클라우드 축과 정렬하는 또 다른 이유는 빈 주위에 ROI 상자를 설정할 수 있기 때문입니다. 그런 다음 ROI를 기반으로 포인트 클라우드를 잘라서 빈 콘텐츠의 포인트만 얻을 수 있습니다.
팁
Smaller point clouds are faster to capture, and can make the detection faster and total picking cycle times shorter.
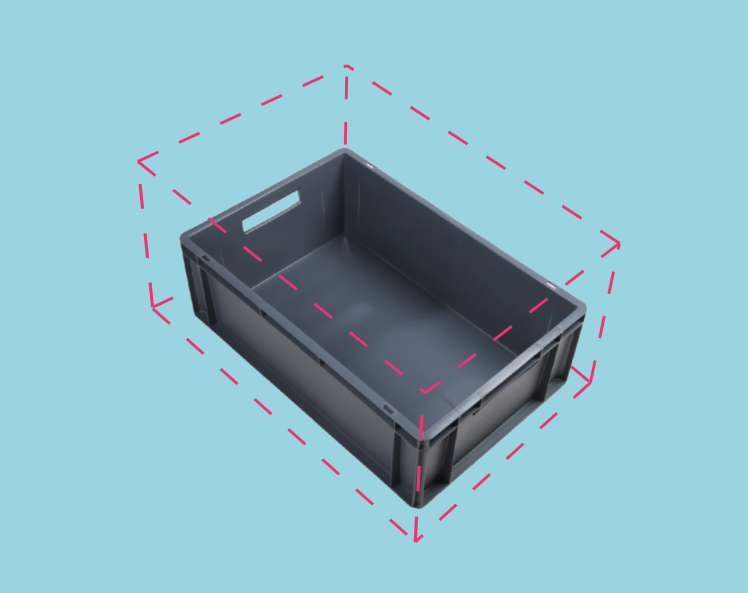
구현 예시는 ROI Box via Checkerboard 을 확인하십시오. 이 튜토리얼은 체커보드에 상대적으로 주어진 ROI 상자를 기반으로 Zivid calibration board 사용하여 포인트 클라우드를 필터링하는 방법을 보여줍니다.
또한 체커보드 대신 ArUco 마커를 사용하는 ROI Box via ArUco Marker 튜토리얼도 제공하고 있습니다.
Stitching
포인트 클라우드 스티칭에는 종종 포인트 클라우드를 다른 좌표계로 변환하는 작업이 포함됩니다.
Multiple stationary cameras
Zivid Multi-Camera Calibration 여러 카메라의 포인트 클라우드를 카메라 중 하나인 마스터 카메라의 좌표계로 변환할 수 있습니다. 다중 카메라 보정에서 스티칭된 포인트 클라우드를 객체 좌표계와 같은 다른 좌표계로 변환하려는 경우가 많습니다.
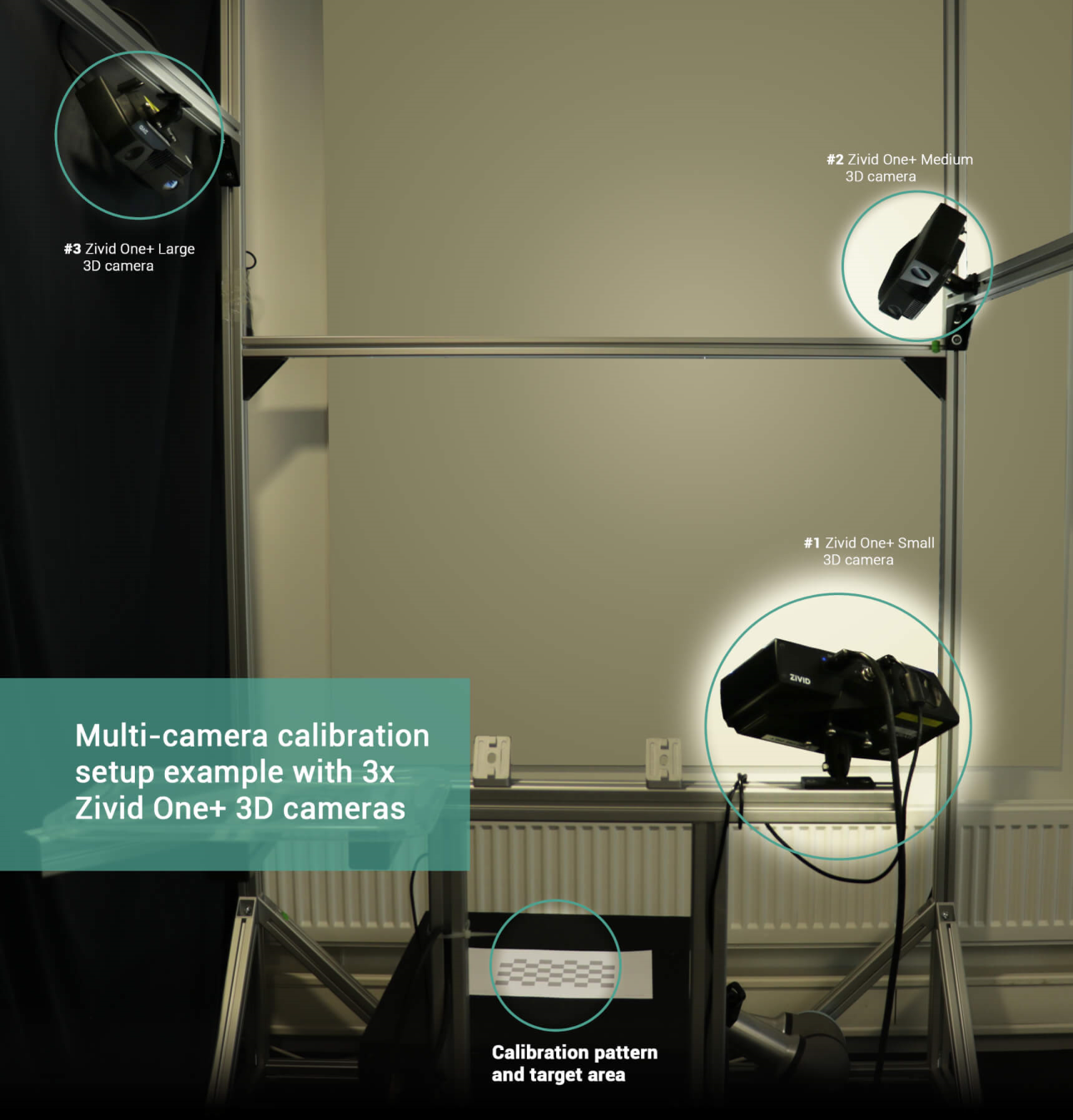
Single robot-mounted camera
로봇 장착 카메라 구성을 통해 여러 뷰에서 동일한 카메라로 찍은 여러 포인트 클라우드를 스티칭할 수 있습니다. 마스터 카메라 좌표계에서 포인트 클라우드를 스티칭하면 객체 좌표계로 변환하는 것이 편리합니다.
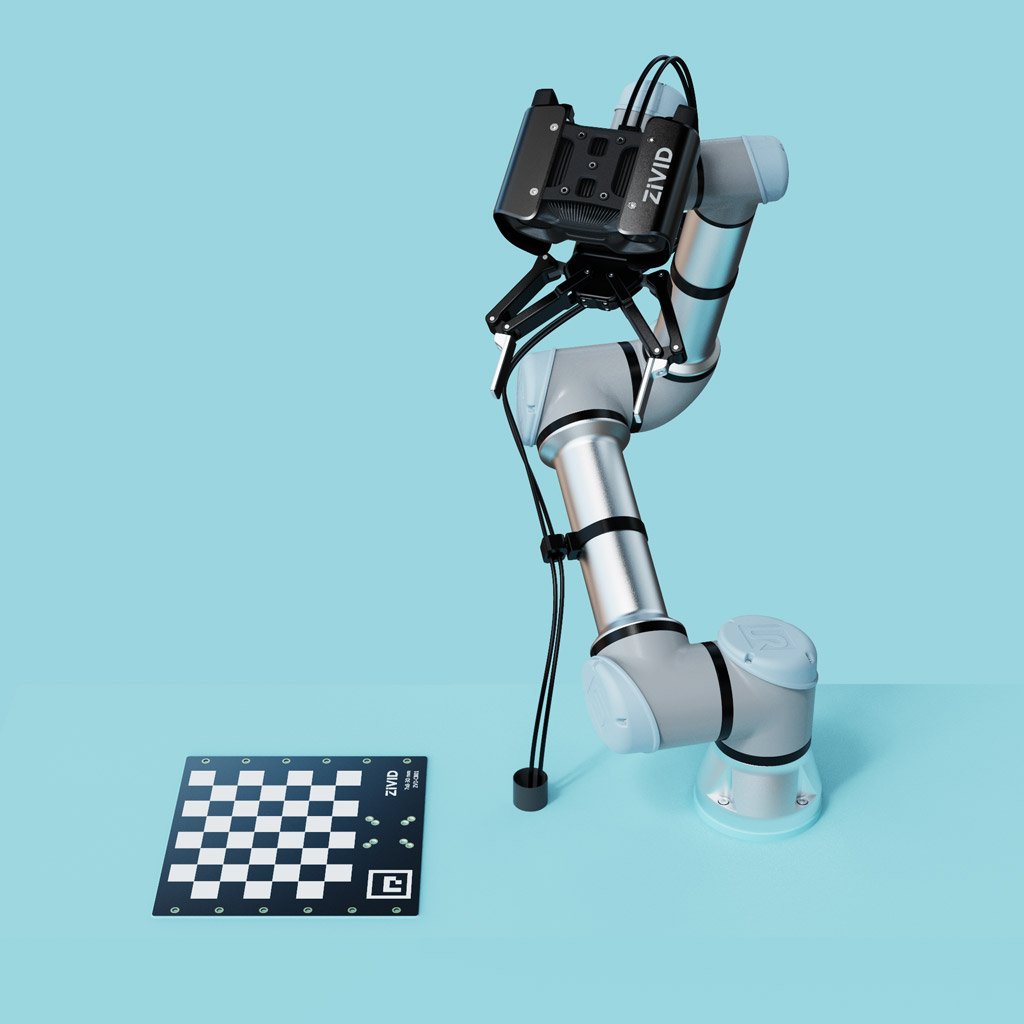
Single camera and turntable
카메라에 대한 턴테이블의 포즈를 알고 있다면 포인트 클라우드를 연결하여 턴테이블 좌표계로 변환할 수 있습니다.
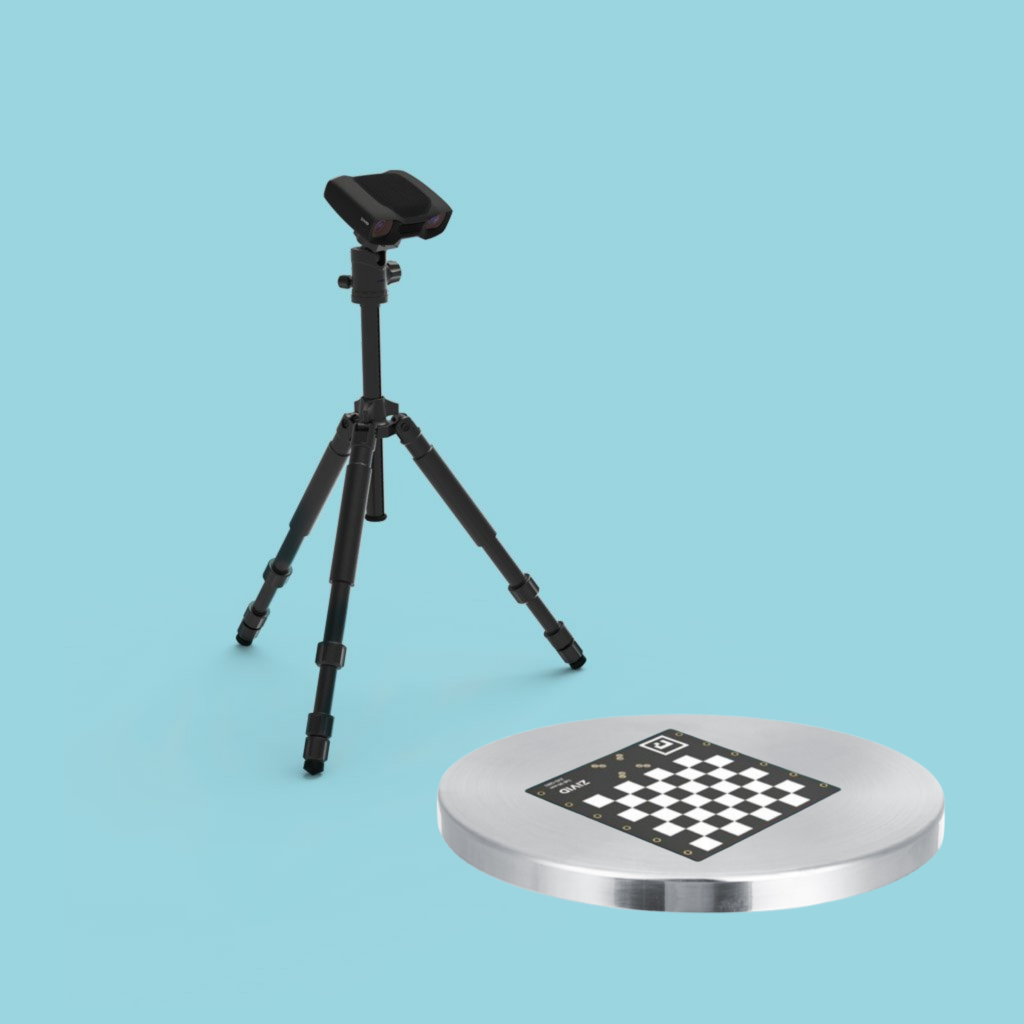
참고
Multi-Camera Calibration 는 SDK의 일부이며 Zivid calibration board 를 사용합니다.
Pose estimation
로봇 베이스 프레임을 기준으로 카메라의 포즈를 가져오는 것은 Hand-Eye Calibration 에 의해 수행됩니다. 임의의 사용자 정의 좌표계와 관련된 포즈가 필요한 경우 기준 마커 및 칼리브레이션 보드와 같은 유용한 액세서리를 장면에 배치할 수 있습니다. 우리는 그들의 기하학을 알고 있기 때문에 그들의 자세를 추정하는 것은 비교적 간단합니다.
구현 예시는 Transform via Checkerboard 을 확인하십시오. 이 튜토리얼은 체커보드의 자세를 추정하고 4x4 Homogeneous transformation matrix를 사용하여 포인트 클라우드를 체크보드 좌표계로 변환하는 방법을 보여줍니다. 또한 Transform via ArUco marker 튜토리얼 있으며, 이 튜토리얼에서 체커보드 대신 ArUco 마커를 사용하여 위치를 특정합니다.
Version History
SDK |
Changes |
---|---|
2.15.0 |
Transform in Zivid Studio is added, with API for getting the currently applied transformation matrix. |
2.0.0 |
Transform API가 추가되었습니다. |