Downsampling Theory
Introduction
이 문서에서는 Zivid SDK를 사용하지 않고 Zivid 포인트 클라우드를 올바르게 다운샘플링하는 방법을 설명합니다.
참고
Zivid SDK v2.1.0부터 다운샘플링이 API에서 구현됩니다.
SDK를 통한 구현에 관심이 있는 경우 Downsample 으로 이동하십시오.
포인트 클라우드를 다운샘플링하기 위해 모든 두 번째, 세 번째, 네 번째 등의 픽셀을 초기의 구성된 포인트 클라우드에서 유지하고 다른 모든 픽셀을 삭제하기로 결정할 수 있습니다. 그러나 Zivid 카메라의 경우 이것이 최선의 솔루션은 아닙니다. 그 이유는 우리의 카메라 센서가 Bayer filter mosaic 가 있기 때문입니다. 4 x 4 이미지에 대한 Bayer 필터 모자이크의 그리드 패턴은 아래 그림에 나와 있습니다. 카메라 이미지의 각 픽셀은 모자이크의 하나의 컬러 필터에 해당합니다. 그리드 패턴의 숫자는 이미지 좌표를 나타냅니다. API에 있는 것과 같은 일부 이미지 좌표 시스템은 (0,0)으로 시작합니다.
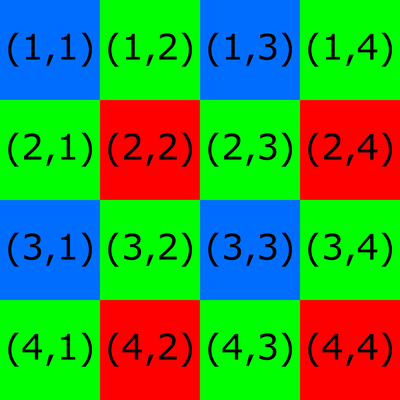
다른 모든 픽셀을 유지하는 다운샘플링 알고리즘을 고려하십시오. 유지된 모든 픽셀은 4가지 가능한 옵션 모두에 대해 동일한 색상 필터에 해당합니다.
(1,1) (1,3) (3,1) (3,3) → Blue
(2,1) (2,3) (4,1) (4,3) → Green
(1,2) (1,4) (3,2) (3,4) → Green
(2,2) (2,4) (4,2) (4,4) → Red
데이터 품질을 유지하려면 포인트 클라우드를 다운샘플링하는 동안 모든 픽셀을 고려해야 합니다. 또한, 다운샘플링된 이미지의 모든 픽셀은 원본 이미지의 짝수 픽셀 격자(2x2, 4x4, 6x6 등)에 대해 수행된 절차에 따라 얻어야 합니다. Zivid 포인트 클라우드를 다운샘플링하기 위한 권장 절차는 다음과 같습니다. 예를 들어 이미지를 축소하여 포인트 클라우드 크기의 절반을 목표로 가정합니다. (예: 4x4에서 2x2)
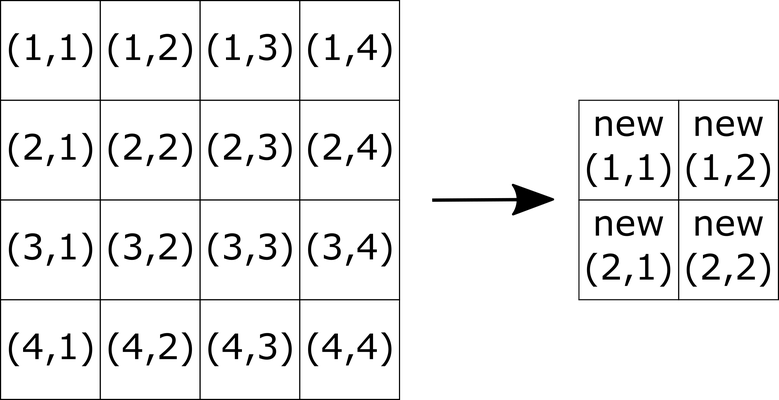
Downsampling RGB values (color image)
새 이미지의 각 픽셀 값은 초기 이미지의 모든 2x2 픽셀 격자에 대한 평균으로 계산해야 합니다. 이것은 각 채널 R, G 및 B 에 대해 수행되어야 합니다. 다음은 새로운 R 을 계산하는 예입니다.
G 및 B 값에 대해서도 동일한 작업을 수행해야 합니다.
R, G, B 값을 계산하는 데 사용되는 것과 동일한 절차를 사용하여 X, Y, Z 를 계산할 수 있습니다. 새로운 다운샘플링된 포인트 클라우드의 값. X, Y, Z 값을 계산할 때 포인트 클라우드의 NaN 값도 처리해야 합니다. 따라서 이전 방법은 최적의 솔루션이 아니며 더 나은 방법은 아래에 설명되어 있습니다.
Downsampling XYZ values (point cloud)
X, Y, Z 새 이미지의 픽셀 값은 초기 이미지의 모든 2x2 픽셀 격자를 가져와서 계산해야 합니다. 각 좌표는 일반 평균 대신 SNR 가중 평균 값을 사용해야 합니다. Zivid가 SNR을 사용하는 방법을 살펴보려면 SNR page 를 확인하십시오.
픽셀의 X, Y, Z 좌표는 NaN 값을 가질 수 있지만 해당 픽셀의 SNR 은 NaN이 아닌 경우가 있습니다. 픽셀이 좌표 중 하나에 대해 NaN 값을 갖는지 확인하기 위해 기본 검사를 수행하여 이를 처리할 수 있습니다. X, Y, Z 좌표 중 하나에 대해 NaN 값이 있는 픽셀이 있는지 확인합니다. 그렇다면 해당 픽셀의 SNR 값을 0으로 바꿉니다. 이것은 예를 들어 다음과 같은 픽셀을 선택하여 수행할 수 있습니다. Z 좌표는 NaN이며 SNR 값을 0으로 설정합니다.
여기서 \(isNAN()\) 은 입력 좌표가 NaN인 픽셀만 선택하는 논리 마스킹 함수입니다.
다음 단계는 모든 2x2 픽셀 그리드에 대한 SNR 값의 합계를 계산하는 것입니다.
그런 다음 초기 이미지의 각 픽셀에 대한 가중치를 계산합니다.
\(NaN \cdot 0 = NaN\) 대신에 \(NaN \cdot 0 = 0\) 으로 처리해야 하는 상황을 피하기 위해 다음을 수행하는 것이 좋습니다.
마지막으로 X, Y, Z 좌표 값을 계산할 수 있습니다. 다음은 새로운 X 를 계산하는 예입니다.
Y 및 Z 값에 대해서도 동일한 작업을 수행해야 합니다.
샘플 코드에서 기본 개념은 동일하지만 작업 순서는 위와 약간 다릅니다.